

- #Granger causality how to
- #Granger causality series
- #Granger causality free
- #Granger causality crack
I can see from the autocorrelation plots, for example, that $Y$ is reasonably smooth but has no other particularly strong internal structure, whereas $X$ has an oscillation with a peak-to-peak period of about 120 time steps (because it is negatively correlated with itself at about 60 time steps).When time series X Granger-causes time series Y, the patterns in X are approximately repeated in Y after some time lag (two examples are indicated with arrows). Thus these plots show the time structure of the strength of the linear relationships both internally (auto) and from one to the other (cross). The cross-correlation function is just the correlation of one time series versus a lagged version of the other, and the auto-correlation is just the cross-correlation of a function and itself. In order to understand what is being plotted, it's first necessary to understand correlation as a measure of the linear relationship between two random variables. The plots of the auto-correlation and cross-correlation functions provide a rough graphical equivalent to the t-tests used in the testing procedure.
#Granger causality how to
I'm also not sure how to interpret the CCF graph The F-test would compare a model with just $X_1$ (or just $X_2$) with the model containing both and find no evidence of improved prediction in the larger model. One can imagine predicting $Y$ with two predictors $X_1$ and $X_2$ where $X_2$ is just $X_1$ with a bit of added noise. The point of the F-test is that it checks that the lagged values of $X$ jointly improve the forecast of $Y$ (or vice versa). (Personally I'm a Jaynesian, but let's not get into that.) Alternatively, you can take the Neymanian view: you don't assert the truth of the null you just choose to act as if the null were true. You can "fail to reject the null" - that is, you didn't find evidence that would warrant rejecting the null. Technically, in the test of $X = f(Y)$ you can't "accept the null". Warning: esoteric bullshtatistical blathering follows. 76 allows me to accept the null for X = f(Y) For example, if there is a variable $Z$ that causally influences both $X$ and $Y$, then the conclusion that $Y$ Granger-causes $X$ can be explained as the influence of $Z$ being felt in $Y$ before it's felt in $X$. If this assumption is not satisfied then Granger-causality is actually Granger-usefulness-for-forecasting. The really important thing to know in terms of key insights is that Granger-causation is only equivalent to causation (in the more common use of the term) under a fairly restrictive assumption, viz, that there are no other potential causes. The fact that first hypothesis was rejected and second was not means that you can use $X$ to forecast $Y$. I really appreciate it if any of you who are well versed with Granger-causality could let me know if I'm interpeting this correctly and also fill in some of the blanks.Ĭaveat: I'm not particularly well-versed in Granger causality, but I am generally statistically competent and I have read and mostly understood Judea Pearl's Causality, which I recommend for more info. I'm also not sure how to interpret the CCF graph.
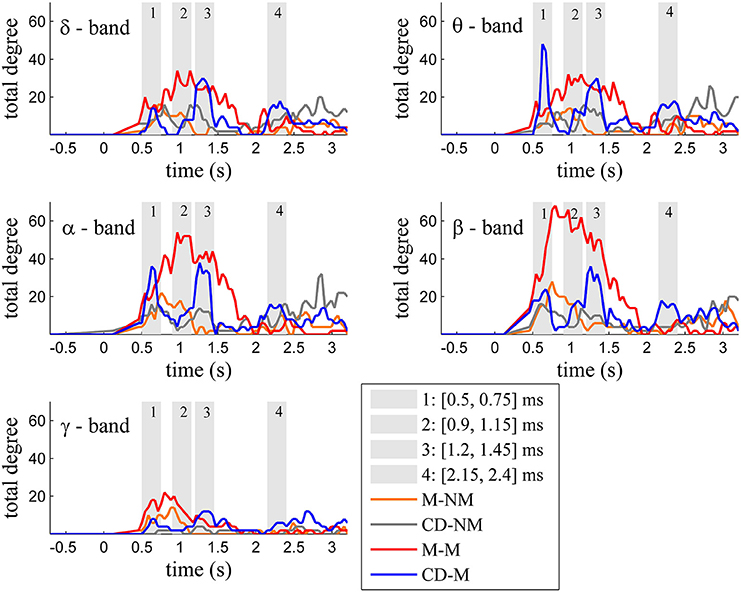
I'm a little rusty on my F-test so I don't really have anything to say on this for now.The fact that first hypothesis was rejected and second accepted is a good thing.The p-value of 0.0000294 means I can reject the null hypothesis that x does not cause y for the Y = f(x).Test was based upon 357 data points and was performed with a lag value of 1.Here are the results and plots that I have interpreted: Summary of computational transaction Also what is the meaning and interpretation of the CCF charts? (I'm assuming CCF is cross correlation.).Is my interpretation directionally correct?.
#Granger causality crack
I have also taken a crack at interpreting the results. Below, is the output from the sample data included on the site.
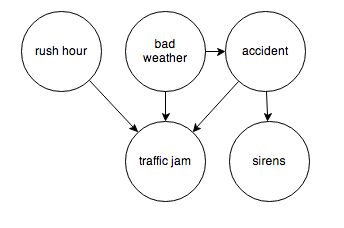
#Granger causality free
I also came across a very helpful tool, the Bivariate Granger Causality - Free Statistics Calculator, that allows you to enter your time series and calculate the Granger Stats. I've read the posts on this site and several good articles online. I'm trying to educate myself on Granger Causality.
